Averaging + Learning: Deterministic and Probabilistic aspects
How do individuals learn when observing others? Canonical models postulate simple models: agents update their own beliefs after observing others. When interacting and learning, players act simultaneously or take turns. Previous models treat learning and interaction to be the same process. But in reality, these two concepts are quite different. Learning can be internal while interaction is a visible external process. We propose several new models of social learning. Our new framework accounts for news and randomness. The connection between discrete and continuous time dynamics is explicitly constructed. With random interaction, convergence in distribution is not always guaranteed and may even lead to peculiar behavior. Although these topics have been studied before, not much has been done to unify them into a single body of work. This work presents a holistic coherent framework. Once we have decided to model reality a certain way, we rigorously develop a theoretical foundation. Suppose individual i is learning about previous estimates of a stock price. She may consider to update her current belief of the stock price as an iteration:
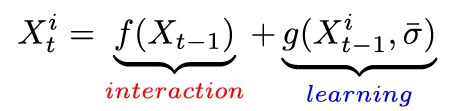
where f is an interaction function, g is an appropriate learning function on some equilibrium value and X_t is the vector of previous beliefs of n agents at time t. If all agents update, will they reach consensus asymptotically? If there is noise in the system, will consensus ever be reached or will the system converge in distribution? Can agents stop learning for short periods and still be wise asymptotically? These questions will be answered by studying possible functional forms for f and g. While discussing theoretical results, we will also try to give intuition and context. The talk will be divided into three sections. Part one will consider the deterministic iterations. In the second part, the discrete time dynamics will feature different types of randomness. Surprisingly, we are still able to construct central limit type of theorems. Finally in part three, continuous time dynamics will be considered with connections to statistical physics.
Links to the seminar recording and the slides.
Last Updated Date : 28/04/2022